
A research team from the University of Eastern Finland has developed MV-DEFEAT, an AI algorithm, to enhance breast cancer screening accuracy.
The AI algorithm holds the potential to transform radiological practices by improving mammogram density assessment.
It utilises deep learning techniques to evaluate multiple mammogram views simultaneously, closely mirroring the decision-making process of radiologists.
Precise mammography interpretation is essential for breast cancer screening, but obstacles such as inconsistent radiological assessments and a global radiology deficit make this difficult.
The research team, including doctoral researcher Gudhe Raju and senior researcher Hamid Behravan, employed a multi-view deep evidential fusion approach, as part of a study.
This method combines elements of the Dempster-Shafer evidential theory and subjective logic to provide a comprehensive analysis of mammogram images from multiple views.
How well do you really know your competitors?
Access the most comprehensive Company Profiles on the market, powered by GlobalData. Save hours of research. Gain competitive edge.
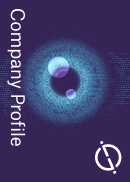
Thank you!
Your download email will arrive shortly
Not ready to buy yet? Download a free sample
We are confident about the unique quality of our Company Profiles. However, we want you to make the most beneficial decision for your business, so we offer a free sample that you can download by submitting the below form
By GlobalDataMV-DEFEAT has demonstrated a 50.78% improvement in distinguishing between benign and malignant tumours over existing multi-view approaches, using the public VinDr-Mammo dataset, which contains more than 10,000 mammograms.
The algorithm’s effectiveness has been consistent across different datasets, indicating its robust performance and adaptability to diverse patient demographics.
Its gradient-based saliency maps highlight regions of interest within the mammograms, aiding radiologists in decision-making.
These results highlight the potential of AI to enhance diagnostic processes, potentially leading to earlier detection and improved patient outcomes in breast cancer care.
Raju said: “To fully integrate AI like MV-DEFEAT into clinical practice, it is crucial to build trust among healthcare professionals through rigorous testing and validation. Indeed, our next steps involve further validation studies to establish MV-DEFEAT as a reliable tool for breast cancer diagnostics in Finland.”