
Artificial intelligence (AI) decision support software is perhaps the most commonly thought-of machine learning technology when it comes to the world of medicine. From diagnoses to discharge planning decisions, these tools can help inform hospitals and clinics – many of which often find themselves stretched to their limits – expediate patient care and, theoretically, provide a better service.
However, patients are not always made explicitly aware of how exactly AI is being used as part of their treatment. Whether an algorithm has been used to help visualise a prostate tumour or to encourage a clinician to talk to them about end of life preparations, the internal machinations of a doctor’s hard drive are rarely the order of the day when discussing the next steps of an individual’s care.
In some cases, this information may be better kept under-wraps – after all, who wants to know end of life arrangements have been brought up with them because a computer suggested it? But in the case of more diagnosis-centric tools, many patients would maintain that they have a right to know what technology is being used as part of their care. What if this information isn’t disclosed, and an AI model makes a faulty recommendation that adversely impacts their health?
The pros and cons of AI in healthcare
IPsoft global practice lead of healthcare and life sciences Dr Vincent Grasso says: “There seems to be broad consensus among medical professionals that AI presents both opportunities and risks that need to be addressed prior to its widespread integration into the healthcare delivery ecosystem.
“The migration from a human workforce to a combined digital/human workforce is underway and thus far has proven to deliver value beyond what was originally expected. However, medical consent requires full disclosure to a patient concerning risks associated with a planned intervention.”
Whether positively or negatively, the risks Grasso speaks of could be heavily impacted by AI, a technology that is far from infallible. It can make wildly incorrect assessments and be littered with racial biases. It often operates very differently in the lab than in real life.
How well do you really know your competitors?
Access the most comprehensive Company Profiles on the market, powered by GlobalData. Save hours of research. Gain competitive edge.
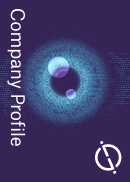
Thank you!
Your download email will arrive shortly
Not ready to buy yet? Download a free sample
We are confident about the unique quality of our Company Profiles. However, we want you to make the most beneficial decision for your business, so we offer a free sample that you can download by submitting the below form
By GlobalDataEarlier this year, researchers from Google Health found that a deep learning system which could diagnose diabetic retinopathy with 90% accuracy under lab conditions caused nothing but delays and frustrations in the clinic. It’s a relatively nascent technology that’s bound to have teething issues but many patients, and a lot of the professionals looking after them, believe they have a right to know when these systems are being used.
Grasso says: “Treatment plans that involve AI should be well thought out by senior leadership. Where newfound risks are identified, plans to mitigate should be constructed. Patients deserve to fully understand all risks related to any healthcare interaction, in order to be best prepared in the event of a mishap.”
AI can be almost as influential as a member of medical staff
Of course, AI doesn’t operate in a vacuum, dishing out diagnoses without any oversight. Clinical AI decision support tools do just that – support, rather than take over, doctor’s decisions. This could arguably make healthcare more ‘human’, by speeding up the time between the first initial doctor’s appointment and disease diagnosis to provide a higher standard of care.
But some decision support algorithms can be almost as influential as human medical staff. Ibex AI, for example, says its Galen platform can help to make up for a shortage of pathologists by taking on the role of a pathologist’s assistant. While patients may be not too concerned about the use of an administrative algorithm, which could do something like save their doctor note-taking time by transcribing their consultation, their reaction may be different when it comes too software with such a significant role.
Future Perfect (Healthcare) clinical advisor and AI lead Dr Venkat Reddy says: “I would use the EMRAD trial for the use of AI for mammography screening as an example. It uses an algorithm instead of a second radiologist for double reporting, with human radiologists having the final say in deciding if the image is typical or atypical. I would expect the use of AI in any future routine clinical screenings like this programme to be made explicit in an information leaflet while taking informed consent from a patient.”
However, many medical professionals would maintain that requesting consent every time a decision support algorithm is used is too time consuming and could potentially derail important conversations about care. Too long may be spent explaining how the AI has come to the conclusions that it, rather than what the final decision means for the patients.
Kearney health and digital principal Paula Bellostas says: “If I’m a patient and a doctor has used an algorithm to either risk stratify me, identify me as a potential patient or even go to the lengths of doing clinical decision support to figure out what the best treatment is for my disease, I’m not sure we should be disclosing.
“As patients, we have never questioned what’s going on in terms of the algorithm that’s sitting inside a doctor’s brain, so why should we now say they need to make explicit the tools they’re using because it’s AI. Also, I don’t believe that any doctor today would take the result of an algorithm without questioning and testing it against their own knowledge.”
Confidence is key
Backlash surrounding algorithmic decision making made headlines in the UK this summer, when students who couldn’t sit their A-level exams due to the Covid-19 pandemic were given a grade by an algorithm instead.
The algorithm didn’t just factor in the academic history of individual students, but the historic grade distribution at the school between 2017 to 2019, leaving nearly 40% of grades lower than teachers’ assessments. It’s not a medical issue, for sure, but serves to highlight a crucial flaw in decision making algorithms across the board – people become very upset when the decisions made by these tools are not seen to be fair.
While the A-levels algorithm was very clearly flawed, its legacy may be something healthcare AI developers ought to keep an eye on.
“With AI in general, there could be some mistrust on the patient’s side,” says Reddy. “There are concerns about current algorithms that are used as symptom checkers in primary care, as the providers can protect themselves from indemnity by declaring that their algorithm is only suggesting possibilities, and not giving a reliable clinical opinion.”
If a patient is denied a treatment they feel they need and they understand that algorithm has been involved in that decision, this could be particularly distressing. As such, it’s vital that medical AI decision support software developers can confidently stand by their product, both inside and outside lab conditions, before releasing it commercially.
Reddy says: “You could argue that it is clinically negligent not to use AI, for example in radiology, if AI and human radiologists together provide better outcomes compared to either of them alone. We need to facilitate shared decision making between patients and clinicians in selecting the treatment options that might involve AI.”