Digital biomarkers can potentially open new doors in clinical research. By using digital sensors or other forms of non-traditional digital means, collected data could validate a patient’s diagnosis, confirm response to an investigational drug, or even predict a patient’s health outcome. Digital biomarkers can also detect changes in clinical measures in specific indications, like movement or neurological disorders.
But there are still many unanswered questions. Collected data can be so vast and granular that it’s not manageable to analyse it via traditional methods, opening an opportunity to employ artificial intelligence (AI). Yet, despite there being a few success stories of AI-generated digital biomarkers, more extensive research is needed to ensure predictive models are safe and accurate.
What defines a digital biomarker is also still not clear. Regulatory bodies have noted that there is a potential misuse of the term “digital biomarker” and the clearance process for software as a medical device (SaMD) is uncharted territory. When developing a digital biomarker, it is important to understand the context of how the data is collected, and the need to correlate outcomes to the indication. Cooperation among multiple stakeholders is needed to ensure the field is not compromised by unproven claims.
Data veracity affects AI-generated digital biomarkers
As monitoring devices rapidly generate a lot of granular data, it can be too complex to understand it visually, Duke Clinical Research Institute chief science and digital officer Dr Eric Perakslis says. For instance, if an accelerometer picks up heart rate at 15 times a second, this level of data detail doesn’t provide much value as such measures are not used in clinical practice.
This unprecedented volume of data puts data analysis pressure on sponsors and CROs. This puts AI under the spotlight as a crucial way to analyse data, which in turn paves the way in digital biomarker development, Perakslis adds.
But first, a reliable and accurate dataset is needed to build and train a predictive AI model, says AI-biotech Insilico Medicine president Alex Aliper, PhD. For example, a machine learning (ML) model, a type of AI, was trained on data from 13 explanatory variables generated within the first 28 days of a 12-week cardiometabolic disease study investigating digital behavioural intervention.
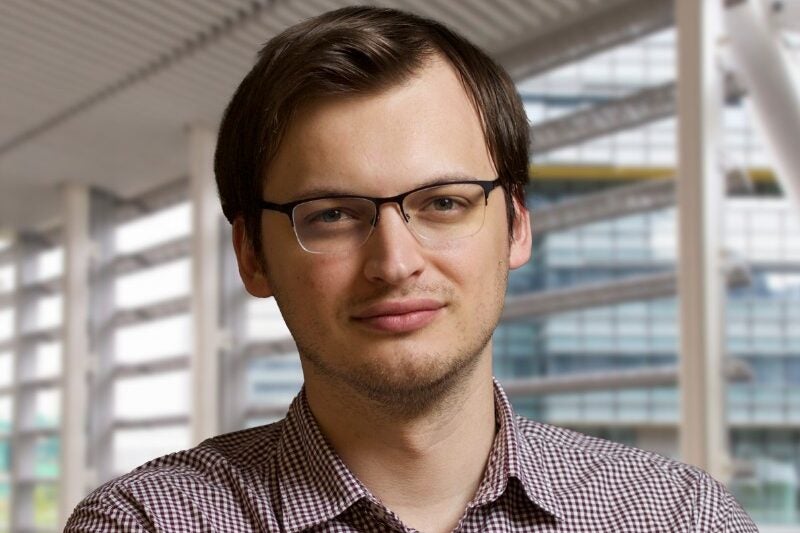
The ML model presented its potential to predict treatment response in participants. However, the authors of the study indicated that a longer training period and a larger dataset is needed to improve the model’s performance. Factors like time since diagnosis, medication adherence, and reliance to self-reported data might also be prone to human error, which would affect the accuracy of generated digital biomarkers.
Data integrity and feasibility are key to building a sufficiently robust and applicable predictive AI model, Aliper says. “If you don’t have the correct measurements, you can proceed in the wrong direction and make a wrong prediction that can be harmful to patients,” he adds. The authors of the cardiometabolic study highlighted that once these AI-generated biomarkers are applied, over-reliance on these digital biomarkers should be avoided, knowing that an unknown source of bias might exist.
Insilico has developed a technology that generates synthetic biological data, creating millions of virtual samples or humans with specific features like age or ethnicity. The generated data is used in the AI training process, especially if the data in specific indication is scarce.
Perakslis noted that, while the current scientific literature on AI and digital biomarkers is positive, there is a lack of success stories from AI companies. “If they were having success, they would be publishing,” he says, adding that scientists need to publish failed studies as well to understand potential pitfalls.
Regulatory framework still a grey area
The definition of a digital biomarker is still not well distinguished and can conflate with an already established clinical outcome assessment (COA), note US Food and Drug Administration (FDA) officials in an article in the journal “npj Digital Medicine” published in March. The FDA defines a digital marker as a characteristic indicating a biological or pathogenic process, or response to an intervention collected by a digital technology.
But the authors raised conflation issues by raising a hypothetical example of using a smartphone to assess hand function. If tap location and time delay was measured and used to identify a neurological disorder, it would be classified as a digital biomarker. But if the tapping task was to measure participant’s functional ability, it would be seen as performance outcome.
Cooperation among regulatory bodies, industry, and non-profits is needed to fully understand what and how digital biomarkers should be used, says Dr Fay Horak, professor of neurology at Oregon Health & Science University. “The more experience and commonality there is, the definitions will crystalise better for the FDA,” she adds. Some pharma companies have already started using secondary or tertiary digital biomarkers in their studies to gain experience, Perakslis notes.
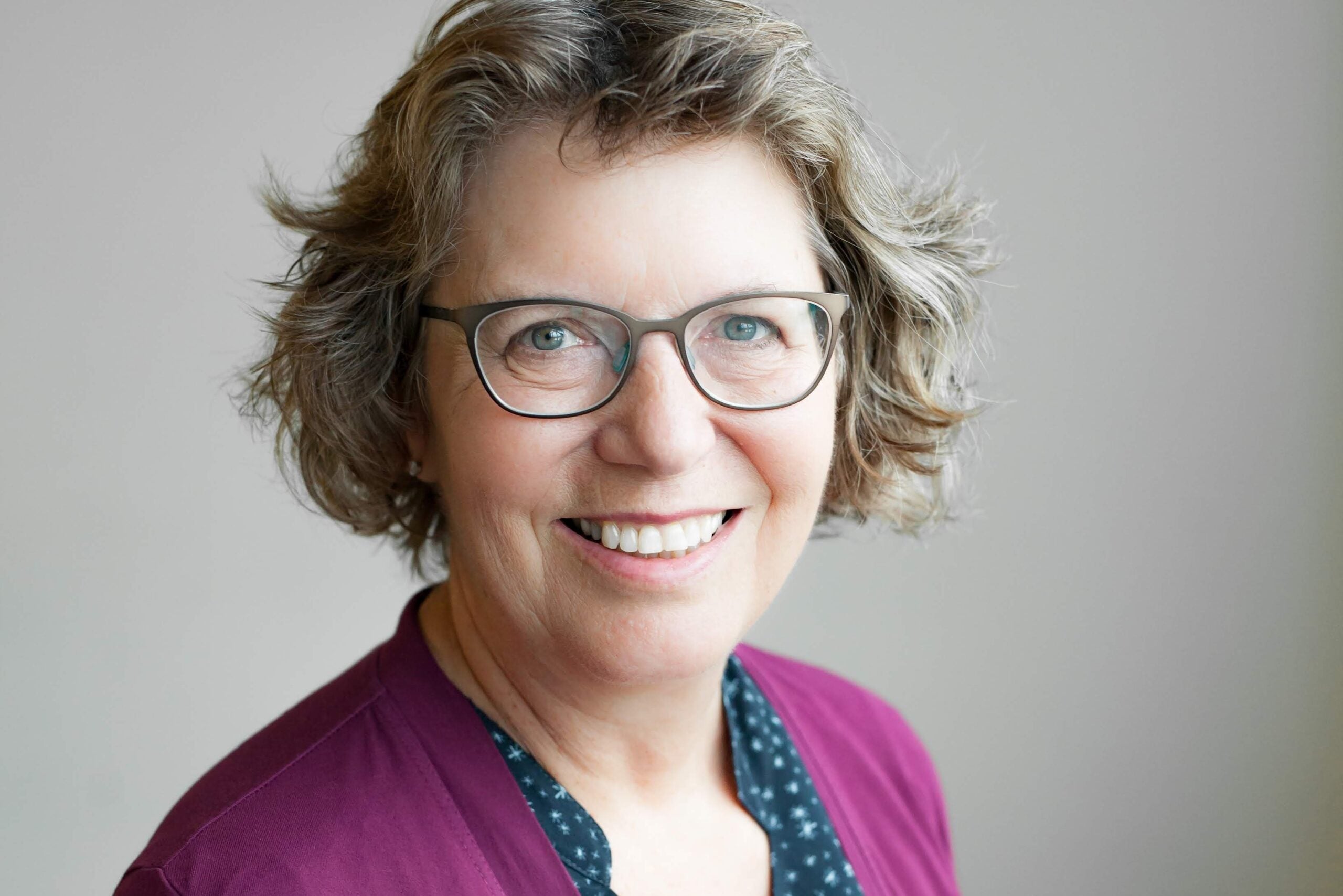
Approving AI-discovered biomarkers faces another set of challenges as they can be regulated as SaMD, says Teresa Arroyo-Gallego, PhD, chief data scientist at nQ Medical. Regulatory agencies are not used to seeing such black-box technologies like AI, she explains. “You can understand AI to some extent but it’s too complicated to comprehend the whole process behind it compared to other medical devices, like scales or thermometers.”
nQ Medical is aiming to get clearance for an AI-powered digital biomarker for early stages of Parkinson’s disease (PD). The FDA has requested to present the algorithm as fixed, meaning it doesn’t change when new data comes in. While it’s not leveraging the full potential of AI, Arroyo-Gallego says that the FDA is not prepared for continuously changing technology and is taking cautious steps.
Challenges in indication specificities and data protection
Digital biomarkers today are in the same position as whole-genome sequencing (WGS) was in its heyday, and it took a decade to find its success in specific indications, Perakslis says. “Just because we have the ability to measure something, it doesn’t mean the thing we are measuring is relevant,” he notes, adding that the industry needs to go through trial and error to fully understand the value of digital biomarkers.
The context in which data is collected to identify a digital biomarker is also crucial, Horak says. If the monitoring device is measuring the quality of walking, different surfaces (like sand or concrete) or activities (such as walking a dog or fast walking) can affect the integrity of the collected data.
Another challenge of using digital biomarkers is the correlation to the studied disease. Horak explains that sponsors need to understand what the most important measure in a certain indication is. For example, the variability of gait should be assessed in ataxia, whereas turn velocity is beneficial in PD. Repeated statistical analyses are needed to ensure that the measured outcomes are appropriate to the specific disease, she adds.
Data privacy and protection is at the forefront with every technology that collects data. One way of assuring patients about their privacy is by collecting or receiving data from healthcare institutions that are “de-identified”, Arroyo-Gallego says. If the data is received directly from patients, sponsors should use blockchain technology in their data infrastructure and store it in isolated and protected entities, like cloud servers. “Data privacy and protection will evolve alongside the development of these tools,” she adds.
Detecting changes in movement
Indeed, while the development of digital biomarkers has yet to be refined, they have had some use in neurological and motion disorders. Wearable sensors can detect miniscule changes in someone’s walking or turning pattern, something that can be missed by a clinician. Monitoring devices are able to detect early signs of a neurological disease showing abnormalities in gait or turning imbalance, even if a neurologist can’t diagnose yet, Horak says.
Sensors allow patient monitoring at home. Horak has developed a smart sock that can fit in a shoe and monitor the movement in the patient’s everyday environment.
The use of telemedicine during the pandemic also bumped up the use of digital biomarkers at home, Horak adds. Telemedicine is one of the most used decentralised approaches, according to Clinical Trials Arena’s exclusive, data-driven Decentralised Clinical Trials (DCT) Adoption Tracker. During telemedicine, the correct use of sensors can be ensured, the patient follows direct instructions and tasks, and the data is received immediately, Horak notes.
Smartwatches can detect patterns
Digital biomarkers powered by devices that track movement, like smartwatches, can be used to detect a pattern, when the patient’s recollection of events can be flawed, Perakslis says. For example, patients with severe colitis or Crohn’s disease might not remember how many times per night they went to the toilet, so a wearable device can be handy.
Arroyo-Gallego advises sponsors to jump into the field of digital biomarkers and start collecting data as secondary or surrogate endpoints. By the time a study is finished, the vast amount of data will be beneficial in continuously training algorithms and helping to uncover new trends. As for companies specialising in AI or digital biomarkers, presenting the product as a full package with context and use cases will be more beneficial than just an idea or a tool. “Don’t give them ingredients, give them a whole meal,” she adds.
The inclusion of digital biomarkers, either generated by AI or not, can reduce the number of participants required in clinical trials and measure changes that are not visible to a human eye. However, it’s not likely that they will replace regular biomarkers in the foreseeable future, Aliper says. Understanding biology is hard and not trivial, and the change must be done gradually and diligently to prove the value of digital biomarkers and performance of AI. Otherwise, unproven claims can compromise the entire field of digital biomarkers, ultimately affecting drug development and patient care.
Takeaways
- AI has the potential to translate complex data to human solvable measures and discover digital biomarkers, but more robust training models need to be used to realise its value.
- Digital biomarkers are still not well defined and can intermix with other measures, like COA. A combined effort among industry, regulatory bodies, and non-profits is needed to crystalise its definition and use.
- In neurological and motion disorders, monitoring devices are effective at collecting miniscule data changes. Also, tracking a patient’s movement can be useful when recollection is easily compromised.