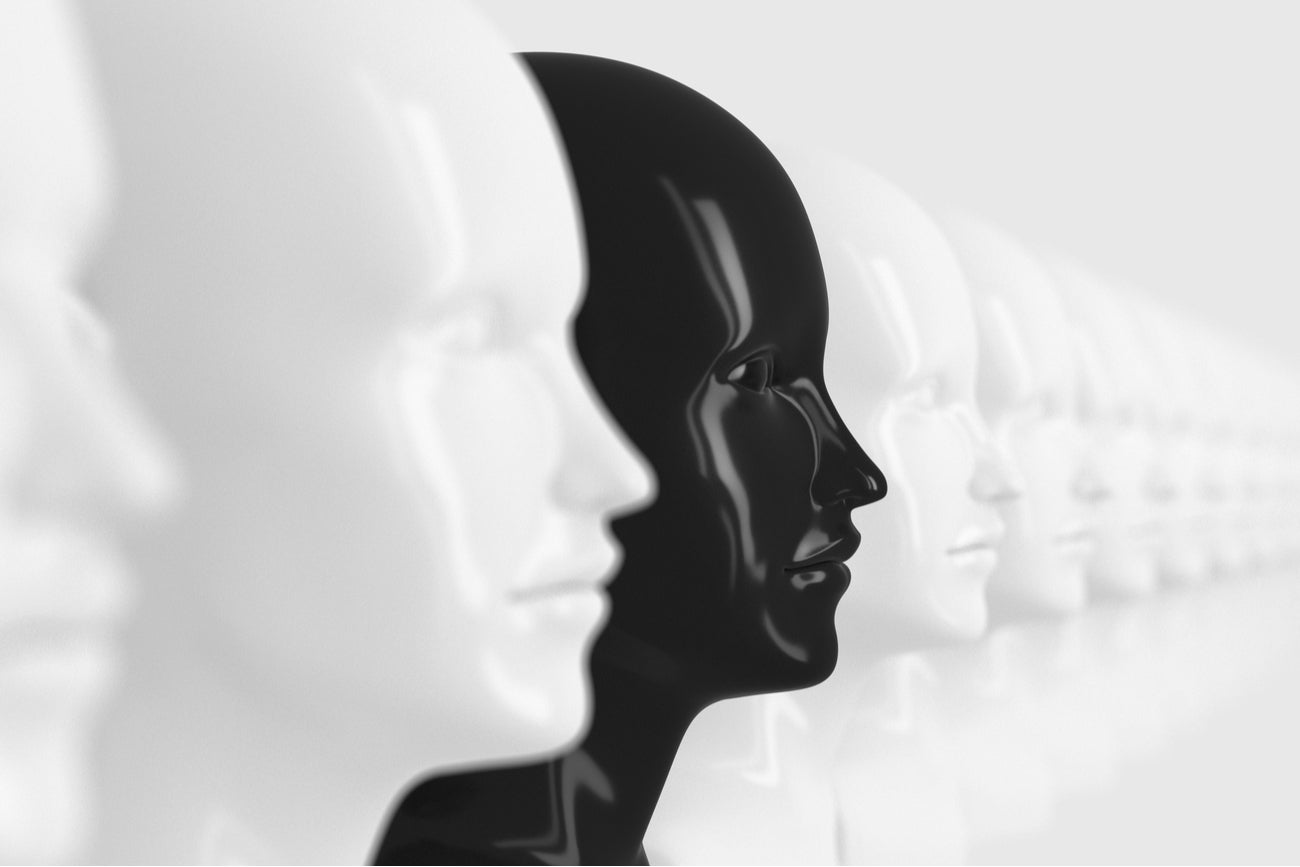
Algorithms and artificial intelligence (AI) are used throughout medicine to assess individual patient risk and guide clinical decisions. From matching organ donation to helping decide how a person should give birth, the software can have a huge impact on the kind of care people receive.
Clinical decision support tools like this can analyse large volumes of data before suggesting next steps for treatment, giving sophisticated insight into the right decisions to make. But a software’s output is only as good as its input.
The medical community still lacks consensus on the bearing that race has over a person’s health, and there is a distinct lack of clear guidelines available on the use of race in medicine. Some physicians maintain that racial and ethnic categories can be reflective of underlying population genetics and so have a practical use in the clinic, and it’s true that certain illnesses are associated with certain demographics.
For example, one in five Irish people carry the gene for haemochromatosis, a medical condition that causes people to absorb too much iron from their diet, and sickle cell disease is thought to be around ten times more common in people with West African ancestry. But mounting evidence suggests that overall, race is not an especially reliable proxy for genetic difference.
Harvard University professor of the culture of medicine David Jones says: “There are places in which precisely defined ancestral populations are biologically defensible and clinically relevant. But you wouldn’t say all white Europeans are at risk of hemochromatosis, just a subset.”
Still, many of these decision-making algorithms adjust their outputs based on patient race or ethnicity, guiding decisions in ways which direct more attention and resources towards white patients than racial and ethnic minority patients.
How well do you really know your competitors?
Access the most comprehensive Company Profiles on the market, powered by GlobalData. Save hours of research. Gain competitive edge.
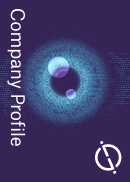
Thank you!
Your download email will arrive shortly
Not ready to buy yet? Download a free sample
We are confident about the unique quality of our Company Profiles. However, we want you to make the most beneficial decision for your business, so we offer a free sample that you can download by submitting the below form
By GlobalData“You’ll see researchers who often pay lip service to the claim that race is a social construct. That said, I think there are still many, many people in the US, especially scientists, who deep down inside believe that race is a biologically meaningful category,” says Jones. “But then they will say that our administrative categories of race somehow correlate with these notions of biogeographic ancestry. And that’s where you really get into trouble.”
Outdated science and erroneous data
A healthcare decision making tool from health services company Optum made headlines in October last year when it was revealed to be inadvertently racially biased. Researchers found that the software, which determined which patients received access to a high-risk healthcare management programme at a US academic hospital, routinely let healthier white people into the programmes ahead of less healthy black people.
The researchers obtained the algorithm-predicted risk score for 43,539 white patients and 6,079 black patients at the US hospital where the software was used. Patients above the 97th percentile were marked as high-risk and automatically enrolled into the health programme, yet black patients were found to have 26.3% more chronic health conditions than equally ranked white patients.
This algorithm had intentionally excluded race from its calculations but used healthcare costs to predict and rank which patients would benefit the most from the programme. Due to structural inequalities inherent in the US healthcare system, black patients access healthcare services less often than white patients do.
The black patients at the hospital spent an average of $1,800 less on healthcare per year than white patients with the same chronic conditions. The algorithm therefore incorrectly assumed black patients would be healthier, as they had spent less money on healthcare.
It’s extraneous factors like these, with sociological rather than biological ties, which can inadvertently lead to algorithmic bias. The Optum developers explicitly attempted to exclude race from their calculations but didn’t consider that a factor used in their algorithm was inherently tied to it.
In a recent article in The New England Journal of Medicine a group of Harvard University researchers, including Jones, reviewed the use of race correction in 13 clinical algorithms used in the US. They unearthed numerous examples of implicit racial bias that made non-white Americans less likely to receive appropriate care.
These algorithms offered rationales for why race was included, based on outdated science or erroneous data which simplistically concluded that poor outcomes for patients of colour were inherently linked to their race.
Race is at once too limited and too broad a category
The decision-making algorithms from the Harvard study also encoded race poorly, with options such as ‘black or nonblack’, ‘describes self as black (fully or partially)’ or ‘white, African American, Hispanic/Latina, Asian American, American Indian/Alaska Native, unknown’. These categories are at once too limited, failing to account for the full expanse of human diversity, and too broad, assuming all people of a certain ancestry will be inherently predisposed to the same health outcomes.
Jones says: “Defenders of the use of race categories will say that in the US we’ve done all this research, and we can find specific alleles in West African populations that are associated with increased risk of certain conditions, so we need to keep race in mind when making clinical decisions because most African Americans have West African ancestry.
“Well, what would you do with Barack Obama? He famously has a white parent and a black parent, so he would be coded as African American by almost any person in the US and that’s certainly how he self-identifies. If he were to have any tests done, he would be counted as black. But his African ancestry is not West African. As Trump continually reminds the American public, his father was from Kenya, and most of these things don’t associate with East African ancestry. So, if you were to apply any of these tools to Barack Obama, you’re making a series of mistakes.”
Jones explains that, if we were to attempt to divide the world into populations based in geographic ancestry, they would be West African, South African and East African. According to what we know of ancient human migration, East African would include not only people indigenous to East African nations, but all Caucasians, Asians and Native American populations.
“Maybe that would be defensible biologically,” he says. “But that would be a really hard sell socio-politically, to tell Donald Trump or Boris Johnson ‘yes, we have new race categories, you are now East African’.”
Race-related disparities in healthcare outcomes exist, not just in the US but globally, and it’s important to take account of them when caring for patients from groups which find themselves disadvantaged.
However, current scientific understanding indicates that those outcomes occur not due to inherent biological factors, but because of the various socioeconomic disadvantages faced by people of colour, such as chronic stress from daily experiences of discrimination, systemic lack of access to resources and even prejudice from healthcare staff.
When socially constructed race categories are incorporated into decision making algorithms, this can lead to the wrong choices being made in individuals’ care as the algorithm incorrectly judges them to be more or less at risk of illness, based on a category with no biological grounding or basis.
Could AI help eradicate racial inequality in medicine?
But what if, instead of encoding racism within medicine, AI could be used to correct negative health outcomes related to race? That’s the belief of Theator co-founder and CEO Tamir Wolf.
Theator uses AI and computer vision to develop a surgical decision-making platform that uses visual AI to scan video footage of real-world procedures. The AI then identifies every key moment during the surgery and annotates it, creating an intelligent, indexed library designed to give surgeons insight into how to improve their performance.
A recent study in the journal Pediatrics has shown that previously healthy black children are three times likely to die or experience complications after surgery than white children.
Wolf believes the first step to closing this gap is to leverage as much real-world surgical data as possible and use AI and computer vision to identify the differences in quality of care that patients receive across racial groups. If these differences can be identified and quantified on a large-scale using AI, then doctors and surgeons will have the educational tools they need to equalise standards of care between races.
“We’re analysing intraoperative surgical footage, and in intraoperative surgical footage a colon is a colon. You can’t discriminate between various races just based on what you see inside the body,” he says. “We analyse that to understand situations and decisions that are being made. Then we can correlate that and connect the dots to see what type of patient went into surgery, connect that with the surgeon and then ultimately what you look at are the outcomes. You connect all the dots along the patient journey and you try to identify patterns that lead to optimal outcomes, ultimately moving to real-time decision support.”
Wolf is speaking specifically about outcomes from surgeries, analysing data about individual procedures on an unprecedented scale to identify racial differences in health outcomes based on aspects of care, rather than chalking them up to some ill-defined but somehow inherent biological factor. This approach may well be generalised to other areas of medicine, but for now surgical decision making is what Theator specialises in.
Of course, in doing do it’s important not to make the kind of mistake Optum did, encoding race within an algorithm accidentally.
Wolf say: “These algorithms are initially algorithms that are created by human individuals, with biases that are either conscious or subconscious and ultimately find their way into the algorithms. But we’re creating algorithms where the focus is alleviating variability and disparity. So everything that we’re doing, we’re conscious of the fact that this is a reality that we need to change. The only way that you can do that is enrich that data set.
“The data has to be as diverse as possible, it to be from thousands of hospitals, it has to be from tens of thousands of surgeons. It has to be with cases that went well and with cases that had errors and complications – you can’t weed these out because you need all of these examples in order to really understand situations and decision making, so that you can ultimately provide decision support that is really meaningful.”
What Wolf is suggesting could well be a viable path to eliminating racial bias in medicine, although it’s immensely ambitious to say the least. But it could be a step towards restructuring society in such a way that racial difference no longer has overarching medical relevance.
It’s crucial, however, to remember that algorithm does not equal neutral or fair, as so many are wont to believe. It is an automation of the ideological framework of the person who designed it.
“If society were truly race blind, for lack of a better word, race would no longer show up as a predictor of medical outcomes, and it could therefore no longer be seen as relevant by expert systems of human-guided data analyses,” says Jones.
“If it were the case that black and white kids had the same survival rate after surgery then the machine learning would just pass right over that and look at different predictors of outcome. Who lives in a slum versus who lives in good housing is likely more salient. But we all have to work towards those kinds of societies. They’re not going to be achieved tomorrow.”