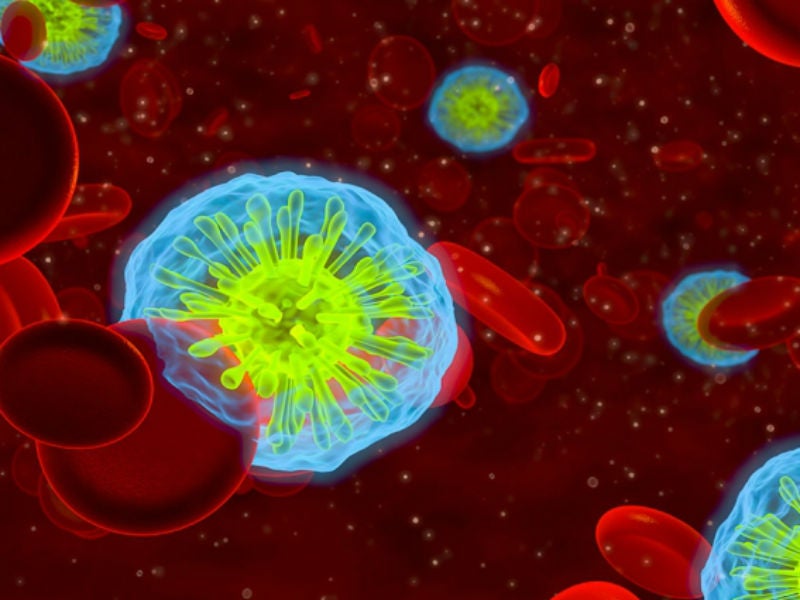
Sepsis is a life-threatening condition that occurs when a patient’s natural bodily responses to an infection go into overdrive and begin to cause harm to tissues and organs. If diagnosed early, the condition is easily treatable with antibiotics. However, sepsis is estimated to cause six million deaths a year around the world and claims a life every 3.5 seconds. The condition is so deadly because it is difficult to diagnose as patients present the same symptoms that they would if they had the flu, gastroenteritis or a chest infection. Unfortunately, a misdiagnosis can often lead to death.
For years, scientists have been working to create a device that would make it easier to diagnose sepsis, and now researchers from Massachusetts Institute of Technology (MIT) and Massachusetts General Hospital (MGH) have developed a predictive model that they think will help clinicians decide when to give potentially life-saving medication to sepsis patients.
Sepsis treatment usually begins with antibiotics and intravenous fluids, administered a couple of litres at a time. If patients don’t respond well then they may go into septic shock and their organs will start to fail. At this point patients will need to go to an intensive care unit (ICU), where clinicians may reduce or stop the fluids and begin vasopressor medications, such as norepinephrine and dopamine, to raise and maintain a patient’s blood pressure.
It is often in the ICU where providing the right treatment to sepsis patients becomes the most difficult. Administering fluids for too long may cause organ damage, so early vasopressor intervention may be beneficial. However, administering vasopressors too early, or when not needed, can cause heart arrhythmias and cell damage. There is no clear-cut answer on when to make this transition so clinicians must closely monitor the patient’s blood pressure and other symptoms, and then make decisions with their best judgement. This is where the MIT team’s new predictive model could really make an impact.
Varesh Prasad, a PhD student in the Harvard-MIT programme in Health Sciences and Technology and first author of the study, explains how the new model could save lives.
Charlotte Edwards: Can you briefly explain the premise behind the predictive model and how it works?
Varesh Prasad: The general idea for this work was to build a system that could aid emergency department clinical staff in determining which patients with sepsis need escalated hemodynamic support – specifically, initiation of vasoactive therapy, i.e. vasopressors. The model does this by making repeated predictions about whether a patient might need vasopressors started at some point in the next few hours. The prediction gets updated every time the clinical staff obtain new information about the patient, such as a new measurement of their vital signs.
We developed this model by extracting data from two years of emergency department visits at MGH. This dataset, which was reviewed manually to help extract import variables, included the patients’ vital signs while in the ED [emergency department], their presenting symptoms and past medical history, laboratory tests ordered in the ED, and interventions performed. We used basic statistical learning methods to determine how the variables included in the data predict need for vasopressors, and then applied this trained model to a separate subset of the data to evaluate its performance.
CE: Why is it useful for clinicians?
VP: The initial presentation of patients with sepsis often belies the severity of the underlying condition: they may look ill, but not critically ill. Their condition may then progress quickly to a state of septic shock, in which blood does not adequately perfuse the vital organs. To help maintain perfusion, the first line of hemodynamic support is to give intravenous fluid. If they get worse, however, they will need vasopressor support.
Research in the past has shown that giving vasopressors in a timely manner is important for patient outcomes in sepsis and also that giving too much IV fluid can be harmful. It is often difficult to tell when to make that transition from using IV fluids to using vasopressors, however, and so the decision is often delayed. In addition, vasopressors must be given through an invasive central line, which takes a significant amount of clinicians’ time to set up in a busy environment, and patients on vasopressors must go to an ICU, so it is also important to not start a patient on vasopressors in the ED unnecessarily.
CE: Could the model be used to aid clinicians in treating any conditions other than sepsis?
VP: The model has been trained for use in patients with sepsis, specifically. The general approach could translate to other acute conditions, but at this time, this model would not be applicable in other clinical settings.
CE: Are there any current drawbacks to the model?
VP: One of the main drawbacks is the challenge in implementation. In particular, to use the model in a new patient, the relevant data have to be entered. Some data, like vital signs, could be drawn automatically from a bedside monitor or a hospital’s electronic medical record system.
Others, however, might have to be first determined by a person and then entered by hand in some way – for example, if the patient has a history of immune compromise. In addition, because the model is only trained on MGH data at this time, we don’t know how well it might work in another setting.
CE: What is next for your team and the predictive model?
VP: This work is part of a larger project in supporting care of patients with sepsis in the emergency department. We are integrating this model with other algorithms to detect who might have sepsis in the first place, as well as other downstream risks related to sepsis, and then to help guide therapy.
We are also working on addressing the implementation challenge by minimising the amount of data a clinician would have to enter by hand at their own discretion to provide the level of support for decision-making that is needed at the bedside at that time.