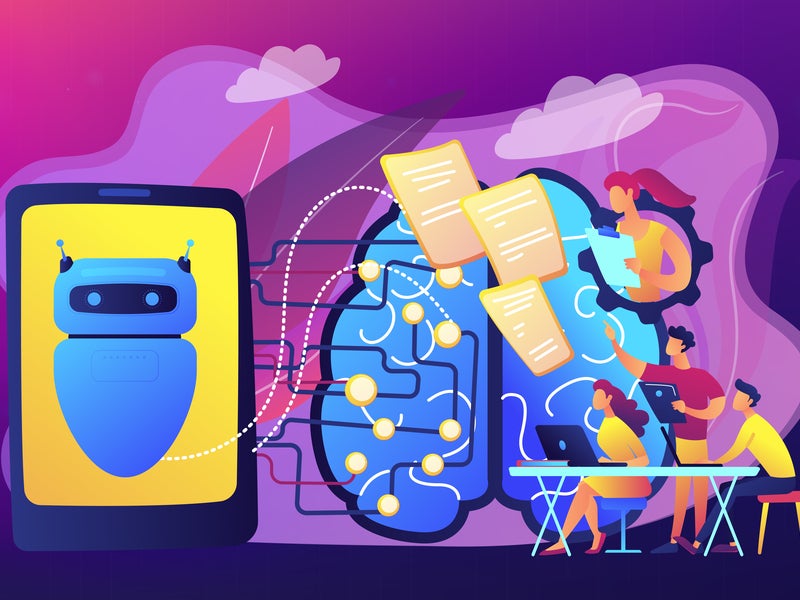
At the beginning of 2017, Amazon’s machine learning division shuttered an artificial intelligence (AI) project it had been working on for the past three years. A team in its machine learning wing had been building computer programmes designed to review job applicants’ resumes, giving them star-ratings from one to five – not unlike the way shoppers can rate products purchased from Amazon online.
However, within a year of the project beginning, the company realised its system was biased against female applicants.
The software was trained to vet applicants by observing patterns in resumes submitted to the company over a ten-year period, the majority of which – due to the male-dominance of the tech industry – came from men. The system therefore taught itself that male candidates were preferable, penalising CVs which contained the word ‘women’s’ and downgrading graduates of all-female universities. By being trained with inherently biased data the machine, in a sense, learned misogyny.
Nearly three years on, interest in AI has only grown, particularly in the healthcare sector. From diagnosis of disease to day-to-day hospital management, the development of artificial intelligence has the power to transform nearly every aspect of healthcare.
AI can give clinicians a combined view of all their data to see where best to deploy their products, and thrives in the imaging field where it can process information from X-rays and scans with a speed and, increasingly, accuracy far beyond humans. The technology has even been used to teach an open-source prosthetic leg to pivot and move based on the wearer’s movements, improving at every turn with new user data.
Questions about the privacy of patient data and the risk of inadvertent discrimination becoming encoded within the technology have left many industry experts concerned about the ethical implications of machine learning. It appears that while the industry is keen to see some official regulatory guidelines on the morality of machine learning in medicine, very few stakeholders are sure what form these should take.
How well do you really know your competitors?
Access the most comprehensive Company Profiles on the market, powered by GlobalData. Save hours of research. Gain competitive edge.
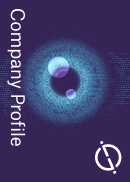
Thank you!
Your download email will arrive shortly
Not ready to buy yet? Download a free sample
We are confident about the unique quality of our Company Profiles. However, we want you to make the most beneficial decision for your business, so we offer a free sample that you can download by submitting the below form
By GlobalDataEthics in AI as business pressure bites
In a survey carried out by the health division of the European Institute of Innovation & Technology (EIT), 59% of healthcare machine learning start-ups expected the AI technologies they were developing to need regulatory approval, but only 22% could suggest topics which should be addressed by guidelines of ethics and AI.
Fortunately for them, the European Commission’s High-Level Expert Group on AI (AI HLEG), a group of 52 independent experts, have published ethics guidelines for trustworthy artificial intelligence.
These guidelines lay out key requirements AI systems should meet to be judged ethical. Big tech companies like Google, IBM and Microsoft have also published their own ethical AI principles – especially handy when many medical companies are using these organisations’ open-access AI solutions to run their own projects.
But as things stand, there’s very little official AI regulation out there, and governments have even been cautioned against attempting to regulate the technology too closely. And when AI is being policed more by guidelines than actual rules, it’s hard to say whether developers of these new technologies will bother taking ethics seriously as a core concern.
EIT Health CEO Jan-Philipp Beck says: “If you’re under business pressure and you need to make sure that you satisfy your investors, are you really going to go all out to make sure you train your algorithm on actually being representative?”
But when an algorithm isn’t trained to be representative, the consequences can be devastating.
Healthcare AI: using (and misusing) data
We think of AI as an arbiter of neutrality, but when fed biased data it churns out biased results.
Siemens Healthineers head of strategy, business development and government affairs Sonja Wehsely says: “To promise there is never a bias is impossible. What industry has to do is make absolutely clear that the way that we provide and develop AI applications is not going to let each and every bias be part of the end result.
“The responsibility therefore is not somewhere with politics or regulation, it’s up to us, it’s absolutely the responsibility of industry.”
A prominent AI system that many US clinics were using to determine which patients received access to healthcare management programmes was recently discovered to be routinely letting healthier white people into programmes ahead of less healthy black people.
The algorithm used healthcare costs to determine patient ‘risk’, or who was most likely to benefit from the programmes. Due to the inherent structural inequalities of the US healthcare system, black patients at a given level of health generated lower costs than white patients. This meant the algorithm saw the white patients as more at risk due to their greater healthcare spend, and thus prioritised them, compared with sicker black patients who were given the same level of predicted risk due to lower spend.
Incorporating other variables to predict this variable saw the percentage of black people referred to the program leap from 18% to 47%.
Of course, enrolment in a voluntary healthcare management programme isn’t the most high-stakes error an AI could have made, and the problem was caught and promptly rectified.
“Everything fails,” says antibiotic AI firm Abtrace’s CEO Dr Umar Naemm Ahmad. “AI will fail, like everything else. But if you have robust testing, that’s the way you can demonstrate that this is something that is safe, not to pretend like it won’t have bias.”
But it’s not just improper use of factors within a dataset that can lead an AI to make erroneous decisions, but its overall context as well.
Philips Research vice president Hans Hofstraat says: “Data is worthless if you don’t know how to use it. The data that you are gathering in Germany will not necessarily have any value in developing an application in Kenya.
“You need to have Kenyan data, because the data from elsewhere are not useful for the healthcare system at hand.”
Ethical AI: a tough balance
Alongside a lack of regulation and encoded algorithmic biases, another major ethical challenge facing the healthcare AI is patient data privacy.
The Royal Free London NHS Foundation Trust received a slap on the wrist from the UK Information Commissioner’s Office for its mishandling of sensitive NHS data after it was found that it had supplied AI firm DeepMind with data on 1.6 million British patients without their consent. Despite the fact that this wasn’t an inherent fault of AI, it did a lot to erode patients’ trust in the software.
It seems obvious that patients need to be able to share as much detail as necessary with their clinicians without the anxiety that their data will wind up in an algorithm for someone else to look at, or otherwise be used inappropriately without their consent. But many commentators fear concerns about data privacy could set back vital research projects.
“We are very, very aware of the perils of misuse of data,” said CSIR Institute of Genomics and Integrative Biology director Anurag Agrawal at the World Health Summit 2019. “At the same time, we would not want people with high levels of healthcare access, and their concerns regarding extremes of privacy, to derail the entire process of generating actionable insights from AI and health data for the community. It’s a tough balance.”
Healthcare privacy regulations often don’t cover the way tech companies wind up using health data. The US Health Insurance Portability and Accountability Act (HIPAA), for example, only protects health data when it comes from organisations which provide healthcare services, like insurance companies and hospitals.
As a result, Facebook was able to roll out a ‘suicide detection algorithm’ in late 2017, which gathered data from a user’s posts to try and predict their propensity to commit suicide, and forward them to helplines and other support services if necessary. A seemingly benevolent act, but the fact remains that Facebook gathered and stored mental health data on its users without their consent.
Of course, these incidents tie into larger questions about the ethics of data privacy in general, but AI has amplified the matter in ways unforeseen in previous decades.
Regulations won’t be implemented overnight. Biased datasets will continue to be generated, fed into algorithms, but hopefully caught and snuffed out. Ethical use of data when machine learning requires so much of it to function is only just beginning to be straightened out. There’s no magic wand that can be waved to ensure that AI will always be used in a responsible and ethical manner.
These are conversations we’re only just beginning to have and while AI can do terrible things it can do great things too. For all its intelligence, AI can’t police itself. The responsibility for this, like it or not, lies with human beings.