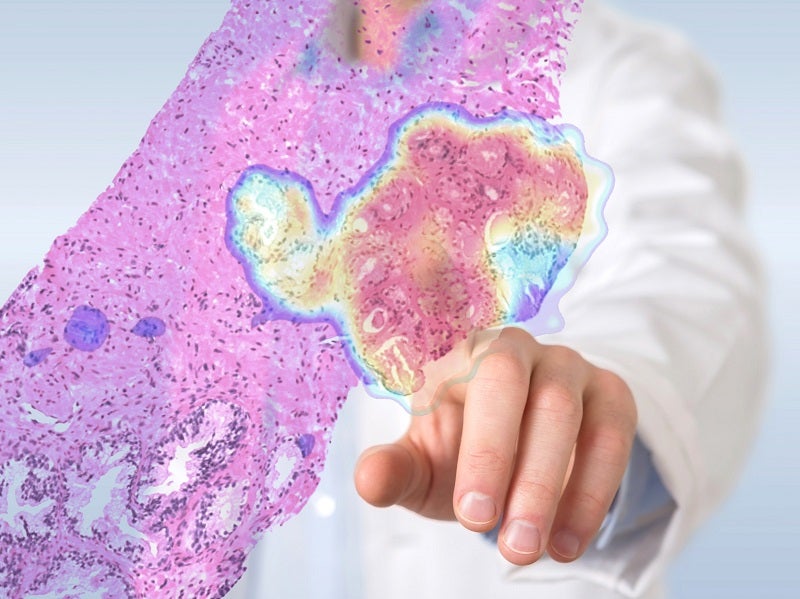
As an aging population prompts a global increase in cancer cases, a decline in the number of pathologists around the world has made diagnoses increasingly difficult to seek. In the UK alone, the shortage of pathologists have led to delays of up to six weeks, all while raising concerns about diagnostic accuracy as individual clinicians are forced to speed through gigantic workloads.
Ibex Medical Analytics is an artificial intelligence (AI)-focused pathology company, which has developed the Galen platform. Galen uses algorithms to analyse images, detect and grade cancer in biopsies and distinguish other clinically relevant factors, in an attempt to help pathologists reduce error rates and work more efficiently.
Researchers at the University of Pittsburgh Medical Center (UPMC) have now found the accuracy levels of Galen Prostate to be the highest reported in the field. The sensitivity measured for prostate cancer detection was 98.46%, specificity was 97.33% and the area under the curve (AUC) was 0.991 – all significantly higher than previously reported metrics for AI algorithms in pathology.
The algorithm also performed impressively when it came to grading the tumours, detecting perineural invasion and tumour sizing, all crucial parts of a pathology report.
Thus far, Ibex has developed the AI powered Galen Prostate and Galen Breast platforms, with more tissue types in the pipeline. These platforms were among the first AI-powered diagnostic systems to become routine in clinical practice. Verdict Medical Devices spoke to Ibex CEO Joseph Mossel to learn more about how the AI works, the impact Covid-19 has had on the company and how it can help make pathology more efficient.
Chloe Kent: How does the Galen platform work?
Joseph Mossel: At its core what it does is mimic the work of a pathologist, so it knows how to accept a scan biopsy, which normally a pathologist will be examining through a microscope. It then knows how to classify the different cell types in the biopsy in the same way a pathologist would do. The platform can grade it, distinguish between different types of cancer and can detect other clinical features which are important but aren’t cancer. Having done that, then you have enough information to generate a pathology report. This is kind of the core technology, using deep learning.
On top of this core technology and the algorithms, we developed two types of the product, First Read and Second Read. First Read is a system which is used by the pathologist while making a diagnosis and it helps them do it faster and with less mistakes, and Second Read is a safety net for pathologists, which raises alerts if it thinks a mistake was done by the pathologist, so this system is about improving the quality of diagnostics or preventing misdiagnosis of cancer.
CK: How did you train the algorithm?
JM: Our strategic partner in Israel is Maccabi Healthcare Services. From them we get the largest bulk of scanned pathology slides that we use to train our algorithms. We have expert pathologists who annotate these slides, mark the different interests and regions of interest and then we feed this into the AI, the deep learning algorithms. It basically then learns how to mimic the work of these pathologists.
You can’t train on data from a single institute, so we have data from partner labs in the US and EU. Every lab that we work with has made some contributions to augmenting our data sets, so it’s already been trained on data from over 10 different pathology institutes.
CK: How does Ibex AI compare to clinicians in terms of accuracy?
JM: It depends on the clinician. In pathology you have specialists and you have generalists, and a lot of the time the general pathologists end up doing a specialist’s work. There, you could see a very high error rate. One of the labs that we’re working with, we saw that for prostate cancer 12% of the cases which they diagnosed as benign were in fact cancer. For me this was a shocking number. Our algorithm maybe misses a cancer one in 1000 cases, so it’s much more accurate than that. We’re yet to find a pathology institute that doesn’t make diagnostic errors, and it’s natural that people make mistakes.
CK: Can technologies like Ibex AI help with the shortage of pathologists?
JM: Up until now we’ve talked about improving diagnostic accuracy, but the other side of it is improving diagnostic efficiency. There’s two ways by which our technology can help achieve that.
One, you can think of it as an assistant working with a pathologist. In advance it already marks which slides are benign and which are cancerous, it marks the interesting regions, it makes some measurements. Just as an assistant doesn’t replace the expert, the algorithm doesn’t replace the pathologist but it gives the pathologist a case which is already pre-cooked.
The other elements where the system can help is that in the practice of pathology a lot of the time what happens is that when the pathologist gets a case for their review, they realise they need some more tests to be run. They send the case back and it can take another day or two or three until they can complete the case. What our technology can do is basically pre-empt this, it can run and then it can pre-order these additional tests that need to be done in a case where it’s important. By the time the pathologist gets the case they already have everything they need to complete the case. It goes beyond just optimising the work of the pathologist, but it optimises the work within the lab.
CK: How has Covid-19 impacted Ibex?
JM: There was definitely an immediate impact in the slowdown in the business but if you look at it in the medium-term and long-term I think Covid-19 is helping people realise how much the move to more digital and AI-based technology is important. It’s become very clear during the Covid-19 crisis there’s a big benefit in being able to work from home when practising pathology. In general we’re seeing a realisation within the healthcare system that ‘normal’ can’t go on and we need an openness for new solutions, a new way of doing things more efficiently and at a better quality.
CK: The Covid-19 crisis has meant far fewer people have been receiving cancer diagnoses as many elective and non-emergency appointments were cancelled. Is Ibex’s AI able to help us play catch up now patients are largely able to see their doctors for routine care again?
JM: We’re seeing exactly this with our customers. For a few months, they were doing nothing, because a lot of these diagnostic procedures are elective and not necessarily urgent, and now they have to deal with this huge bulk of work coming in that they need to do rapidly. The results of doing work very rapidly and this huge workload is that they do it, by nature, at a lower level of quality. Having our algorithms in place guarantees that even though they’re dealing with a huge bulk of work now, they can still maintain the same level of quality that they always have.