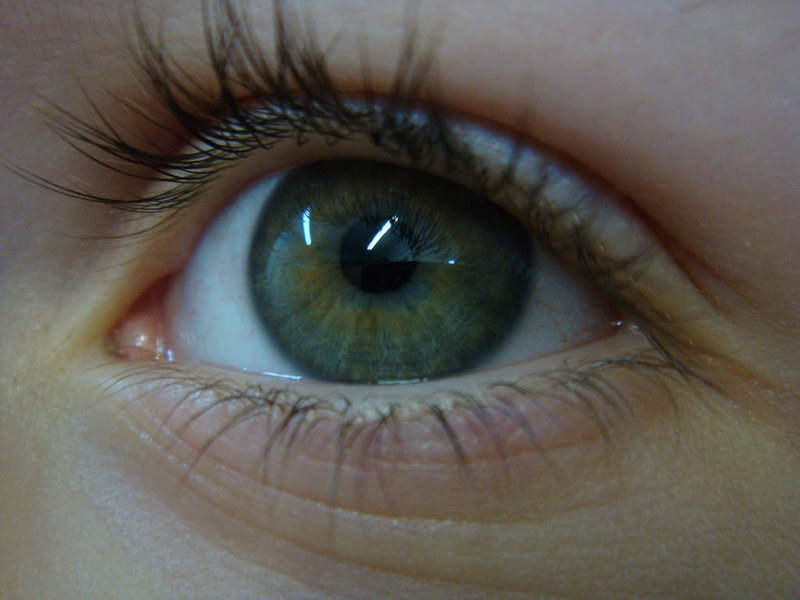
Health researchers at Google have used artificial intelligence (AI) to predict cardiovascular (CV) risk factors through analysis of retinal images.
The new research is based on the team’s previous findings of using deep learning techniques for accurate diagnosis of diabetic eye disease using medical imaging.
During the latest study, it was found that deep learning algorithms, which were trained on data from 284,335 patients, could predict CV risk factors from retinal images with high accuracy for individuals from two separate datasets of 12,026 and 999 patients.
They further observed that the algorithm could detect patients who would develop a major CV event in the future in 70% of the cases. This accuracy is said to be comparable to other CV risk calculators that require a blood test for cholesterol.
In a statement, the team said: “We show that in addition to detecting eye disease, images of the eye can very accurately predict other indicators of CV health.
“This discovery is particularly exciting because it suggests we might discover even more ways to diagnose health issues from retinal images.”
How well do you really know your competitors?
Access the most comprehensive Company Profiles on the market, powered by GlobalData. Save hours of research. Gain competitive edge.
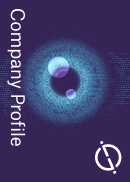
Thank you!
Your download email will arrive shortly
Not ready to buy yet? Download a free sample
We are confident about the unique quality of our Company Profiles. However, we want you to make the most beneficial decision for your business, so we offer a free sample that you can download by submitting the below form
By GlobalDataThe researchers additionally explored the way the algorithm made CV risk predictions to ensure that the approach is reliable and can aid in further research.
Researchers added: “Our approach uses deep learning to draw connections between changes in the human anatomy and disease, akin to how doctors learn to associate signs and symptoms with the diagnosis of a new disease.
“This could help scientists generate more targeted hypotheses and drive a wide range of future research.”
They believe that further studies are required using larger and more comprehensive datasets for validating the CV risk prediction capability of the algorithm.