
Cybersecurity researchers at Ben-Gurion University of the Negev (BGU) in Israel have developed malware to demonstrate vulnerabilities in medical imaging equipment.
As part of the demonstration, the researchers hacked and edited 3D medical scans to add or remove images of tumours. This was performed using a generative adversarial network (GAN) that was trained using medical images that are available for free on the internet.
When the team asked three radiologists to interpret a mixture of altered and genuine CT scans, they misdiagnosed 99% of the scans that had been altered to add a tumour and 94% of those where cancerous cells were digitally removed.
Even after radiologists were informed about the attack, they were unable to distinguish between the altered and genuine images.
The scans were also checked by an artificial intelligence (AI) algorithm, which could not identify the fake images.
Poor cyber protection could lead to ransomware and cyberterrorism attacks on hospital networks. Edited scans like those used in the experiment could be used to commit insurance fraud.
How well do you really know your competitors?
Access the most comprehensive Company Profiles on the market, powered by GlobalData. Save hours of research. Gain competitive edge.
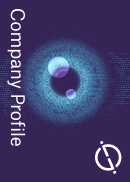
Thank you!
Your download email will arrive shortly
Not ready to buy yet? Download a free sample
We are confident about the unique quality of our Company Profiles. However, we want you to make the most beneficial decision for your business, so we offer a free sample that you can download by submitting the below form
By GlobalDataA researcher at the BGU National Cyber Security Research Center Yisroel Mirsky said: “Our research shows how an attacker can realistically add or remove medical conditions from CT and MRI scans.
“In particular, we show how easily an attacker can access a hospital’s network, and then inject or remove lung cancer from a patient’s CT scan.”
According to the team, a hacker would have complete control over the size, location and number of tumours added to the image.
Mirsky added: “In addition to the radiologists, we also showed how CT-GAN is an effective adversarial machine-learning attack. Consequently, the state-of-the-art artificial intelligence lung cancer screening tools, used by some radiologists, are also vulnerable to this attack.”
The team said that using encryption between the hospital’s radiology network and using digital signatures to authenticate scans could help cut the risk.