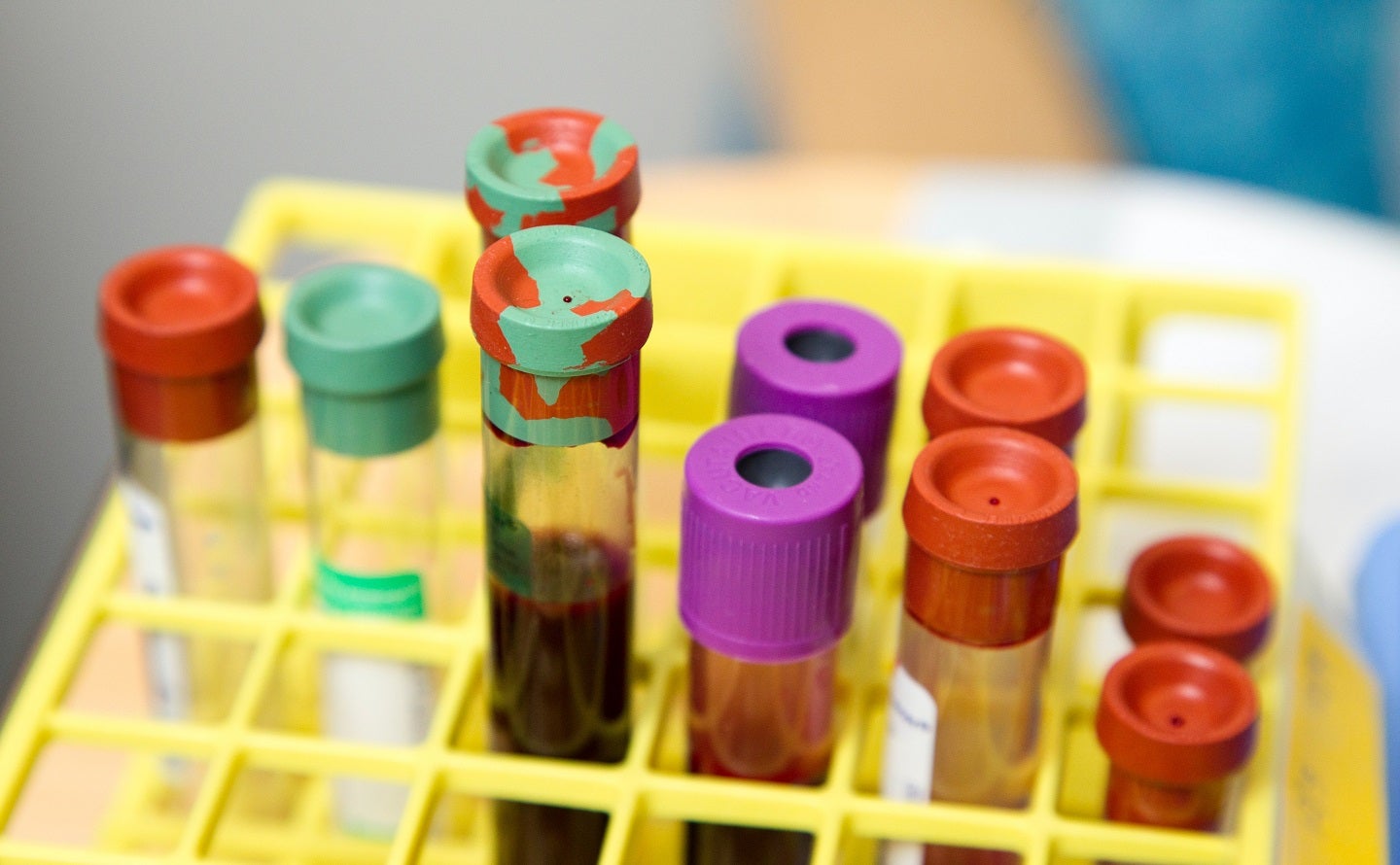
The University of California, Santa Cruz (UC Santa Cruz) Biomolecular Engineering assistant professor Daniel Kim and his lab have developed an RNA liquid biopsy platform, which identifies both protein-coding RNA and RNA dark matter in the blood.
The new approach has been demonstrated to significantly enhance the performance of liquid biopsy for cancer diagnosis.
They are developing more precise liquid biopsy technologies by harnessing signals from RNA’s less explored ‘dark matter’ in the genome.
Kim’s latest research demonstrates the presence of this genetic material in the blood of individuals with cancer. It can be utilised to diagnose various cancer types such as pancreatic, oesophagal, lung and others at an early stage of the disease.
The lab developed an advanced platform named COMPLETE-seq for cell-free RNA sequencing and analysis, designed specifically to detect repetitive non-coding RNAs that are often overlooked.
After drawing a patient’s blood, this comprehensive method examines the sample for both the transcriptome’s annotated areas and the five million non-coding repetitive elements that Kim’s lab focuses on.
How well do you really know your competitors?
Access the most comprehensive Company Profiles on the market, powered by GlobalData. Save hours of research. Gain competitive edge.
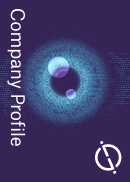
Thank you!
Your download email will arrive shortly
Not ready to buy yet? Download a free sample
We are confident about the unique quality of our Company Profiles. However, we want you to make the most beneficial decision for your business, so we offer a free sample that you can download by submitting the below form
By GlobalDataThe researchers also deployed nanopore sequencing to analyse the cell-free RNAs in the blood, helping to generate long reads and determine the true length of these RNAs.
Kim said: “If you look at these different cancers, each has its own characteristic cell-free RNA profile, but a lot of these RNAs are coming from the millions of repeat elements that are found throughout the genome.
“What we found was that when we trained machine learning models for cancer classification, the models perform better when you introduce these repetitive cell-free RNAs as additional features.”