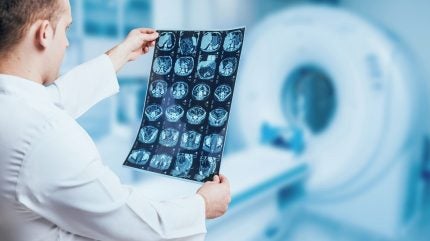
Researchers at University College London (UCL) in the UK have developed MindGlide, an AI tool that evaluates magnetic resonance imaging (MRI) brain images and detects the changes and damage that occur due to multiple sclerosis (MS).
This deep learning model is said to extract important data from MRI scans such as measuring damaged brain regions and emphasising shrinkage and plaques of the brain.
UCL noted that MindGlide was tested in a new trial on more than 14,000 images from over 1,000 MS subjects.
This AI tool can identify the way various treatments impact the progression of the disease in trials and usual care.
It has reduced the time taken to report these images from weeks to mere seconds for each image, a task that formerly required manual interpretation by expert neuro-radiologists.
The study also demonstrated that MindGlide’s performance was better than existing AI tools, SAMSEG and WMH-SynthSeg, by 60% and 20%, respectively, in brain abnormality location and monitoring treatment impacts.
Findings from the study also showed that there’s a possibility to leverage the tool for identifying and measuring key brain tissues and lesions even in cases with “limited” MRI data and single scan types that are not generally intended for this.
It has shown effectiveness in both the brain’s outer layer and deeper regions.
The tool has also corroborated earlier research on the effectiveness of various MS treatments.
While currently limited to brain scans, future developments aim to include spinal cord imaging for a comprehensive evaluation of MS.
UCL Hawkes Institute and UCL Queen Square Institute of Neurology’s Dr Philipp Goebl said: “Using MindGlide will enable us to use existing brain images in hospital archives to better understand multiple sclerosis and how treatment affects the brain.”
MindGlide’s development involved training on an initial dataset of 4,247 brain MRI scans from 2,934 subjects across 592 MRI scanners.
This latest study was conducted to validate the tool against three separate databases of 14,952 images collected from 1,001 individuals.