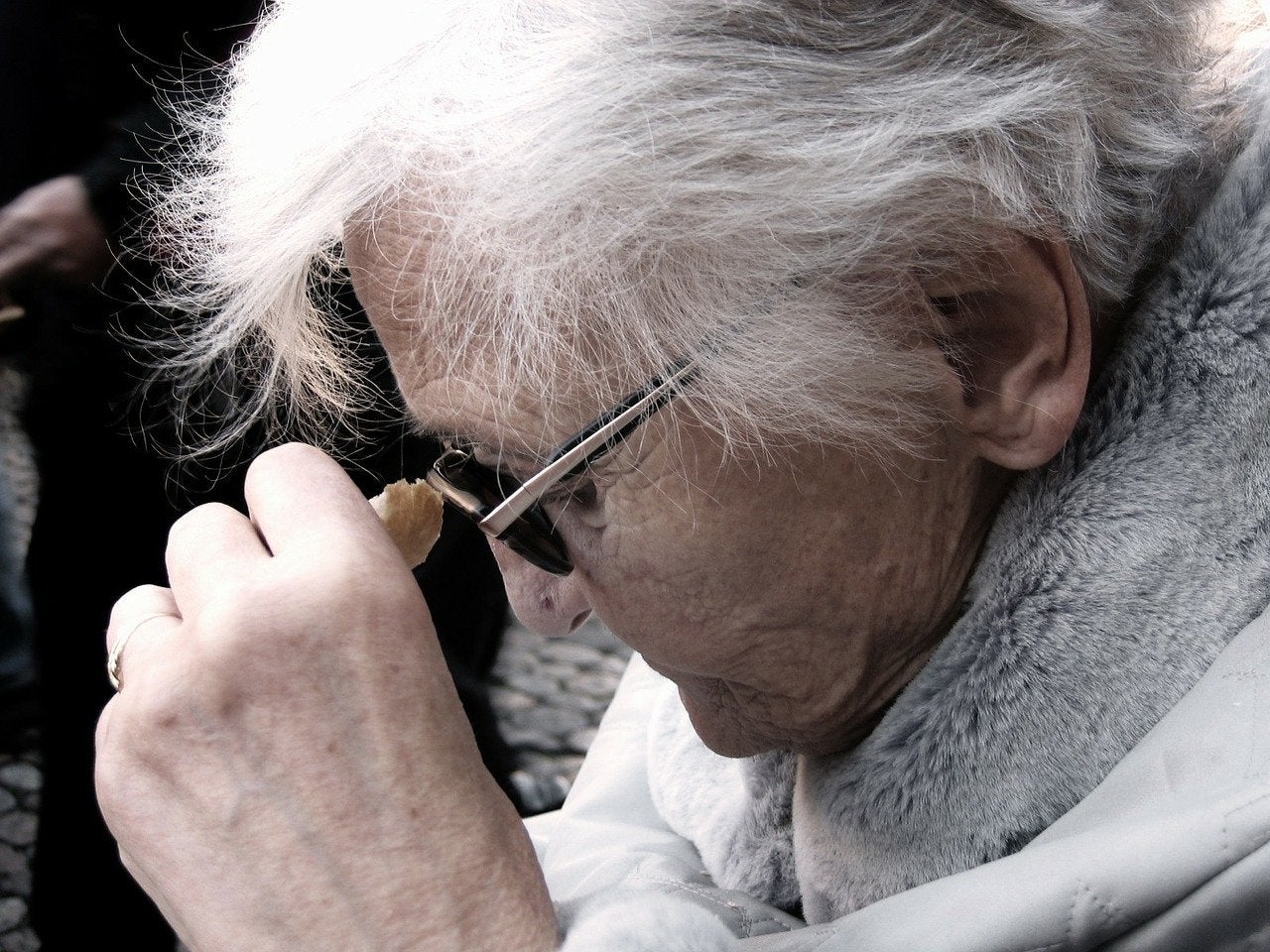
A student team at USC Viterbi School of Engineering in the US has developed an artificial intelligence (AI)-based tool to detect the early onset of Alzheimer’s disease.
The machine learning algorithms use the speech patterns of an individual to diagnose the onset of the disease.
USC Viterbi School of Engineering computer science undergraduate students Leena Mathur and Nisha Chatwani led the research.
The team undertook machine learning research to analyse speech patterns, as well as the choice of words, that could help automatic systems detect the disease.
According to the Alzheimer’s Association, around six million people in the US have Alzheimer’s disease, and it is said to be the sixth-leading cause of death in the country.
Usually, doctors conduct tests such as the Cookie Theft picture test to check memory loss and other thinking abilities.
How well do you really know your competitors?
Access the most comprehensive Company Profiles on the market, powered by GlobalData. Save hours of research. Gain competitive edge.
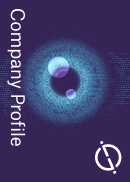
Thank you!
Your download email will arrive shortly
Not ready to buy yet? Download a free sample
We are confident about the unique quality of our Company Profiles. However, we want you to make the most beneficial decision for your business, so we offer a free sample that you can download by submitting the below form
By GlobalDataIn this test, patients are shown a picture and asked to describe what they see. The doctors then analyse their speech patterns to check if a person has Alzheimer’s disease.
However, this process of detection can be expensive and take months to finish.
Furthermore, around 58% of the 44 million people suffering from Alzheimer’s across the world live in less developed countries, where such testing methods are not easily accessible.
Mathur said: “We were inspired to start this project because we found the problem of dementia diagnosis compelling, specifically the development of low-cost, non-invasive and scalable systems that can do this effectively.”
With the new low-cost, AI-based tool, the student team has automated the diagnosis through analysing speech patterns process.
The team collected a dataset of audio clips, as well as transcripts, of 293 patients describing a stimulus image. This dataset was taken from a National Institute of Health study conducted at the University of Pittsburgh.
The team integrated this dataset into the machine learning model.
The tool analysed the speech patterns in the clips and used information from verbal and audio modalities to take clues for diagnosing Alzheimer’s disease.
Mathur continued: “For example, our feature extraction captures aspects of verbal structure that psychologists have linked to analytic thinking, such as the structure and use of prepositions and conjunctions.
“People with Alzheimer’s dementia, while responding to the stimulus photo, leveraged language that was significantly less indicative of analytic thinking. In addition, participants with Alzheimer’s tended to use the past tense significantly more than the control group, which informed our models.”
The team now plans to explore multimodal methods that integrate and sync information drawn from both modalities for a better diagnosis of the disease.